Maelstrom guidelines for retrospective data harmonization were developed by the Maelstrom Research team to ensure quality, reproducibility, and transparency of the data harmonization process. Based on these guidelines, retrospective harmonization is an iterative process involving a series of closely related, interdependent, and often integrated steps (see Figure below).
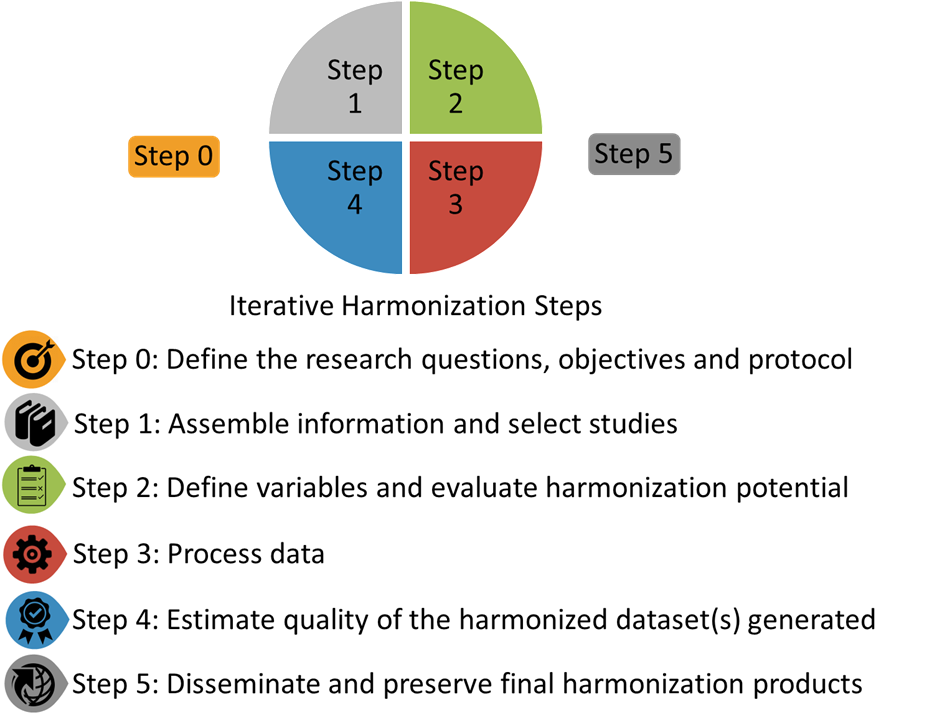
-
Step 0. Define the research question(s), objectives and protocol
Before the harmonization process begins, it is necessary to develop a protocol reflecting the potential and limitations of the collaborative research project and to clearly define the research question(s) and objectives.
-
Step 1. Assemble information and select studies
As a starting point to the harmonization process, it is required to gather appropriate knowledge and understanding of each study. This includes documenting all the relevant individual study characteristics, such as design, and type and format of data. Participating studies should also be selected based on rigorous criteria.
-
Step 2. Define variables and evaluate harmonization potential
To ensure content equivalence of the new (harmonized) variables to be created across studies, these variables must be defined. The group of core variables targeted for harmonization is called the DataSchema. It is important to thoroughly evaluate the potential for each study to construct each DataSchema variable.
Retrospective data harmonization requires to find a satisfactory (scientifically valid) balance between accepting only precisely uniform variables that render pooling straightforward (e.g. exact question or standard operating procedures) but limit the potential to integrate multiple studies; and accepting a certain level of heterogeneity across participating studies providing similar but not necessarily identical data. It is important to note that definitions of DataSchema variables and harmonization potential are context-specific and vary according to a project’s scientific objectives and the level of precision needed for the planned analyses. That is, information considered ‘compatible’ in one project may not be ‘compatible’ in another.
-
Step 3. Process data
To enable data processing, it is essential to ensure that all the study-specific data items required to generate the DataSchema variables are available and that the collected information is valid. The approach used to process data under a common format will vary depending on the variables to be harmonized, the data collected by each study and the possibility to pool data.
-
Step 4. Estimate quality of the harmonized dataset(s) generated
In order to ensure that statistical analyses are conducted on data of acceptable quality, quality control procedures must be implemented. It is essential to evaluate the quality of the harmonized dataset so as to ensure the validity of data analysis results.
-
Step 5. Disseminate and preserve the final harmonization products
As a final step, it is essential to adequately preserve and disseminate the harmonized data and provide access to all documentation needed to understand the quality and content of the harmonized dataset.